Why YouTube Shouldn’t Over-Rely on Artificial Intelligence to Police Its Platform
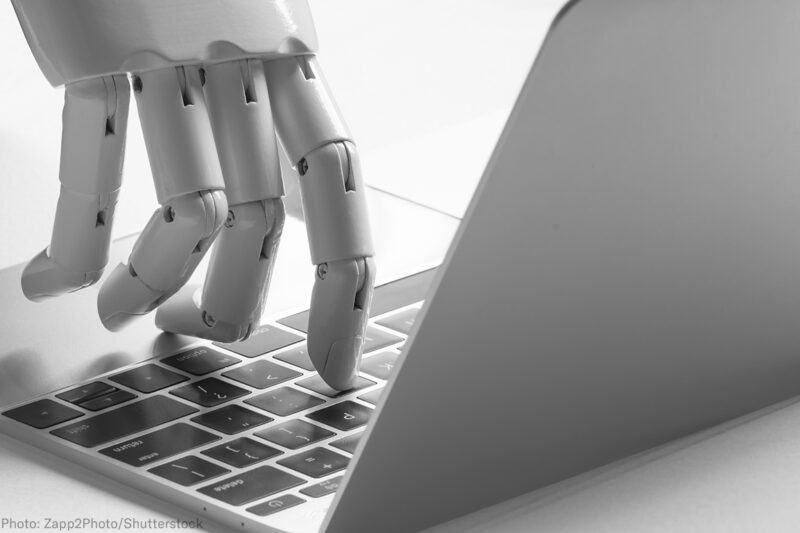
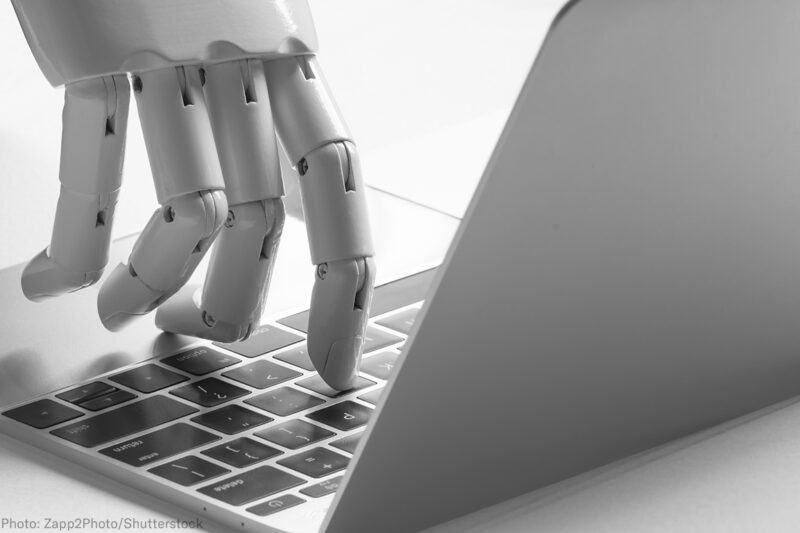
Social media companies are under tremendous pressure to police their platforms. National security officials press for takedowns of “terrorist content,” parents call for removal of “startling videos” masquerading as content for kids, and users lobby for more aggressive approaches to hateful or abusive content.
So it’s not surprising that YouTube’s first-ever Community Guidelines Enforcement Report, released this week, boasts that 8,284,039 videos were removed in the last quarter of 2017, thanks to a “combination of people and technology” that flag content that violates YouTube policies.
But the report raises more questions about YouTube’s removal policies than it answers, particularly with regard to the use of machine-learning algorithms that flag and remove content because they detect, for example, “pornography, incitement to violence, harassment, or hate speech.”
Content flagging and removal policies are increasingly consequential. Because so much speech has migrated onto major social platforms, the decisions those platforms make about limiting content have huge implications for freedom of expression worldwide. The platforms, as private companies, are not constrained by the First Amendment, but they have a unique and growing role in upholding free speech as a value as well as a right.
YouTube’s new report, while an important step toward greater transparency, doesn’t resolve those concerns. First, while it assures that a human reviews content flagged by artificial intelligence, it neither describes the standards for this review process nor reveals how frequently human reviewers reject the machine’s initial flag. This is especially concerning for content flagged as “violent extremist content.” In the last quarter of 2017, a staggering 98 percent of content removed for reflecting violent extremism was flagged by machine, which raises the concern that YouTube may be relying almost exclusively on automated tools to flag content in the first instance. Does YouTube have a robust system in place for determining when algorithmically identified “violent extremist content” actually features violence or incitement to violence? Or does “human review” mean rubber stamping what the machines have labeled terrorist propaganda?
Deciding what constitutes “extremism” is notoriously fraught — under the best of circumstances, it is subjective, political, and context-dependent. The obvious danger is that efforts to police “extremist” content will be arbitrary, will discriminate against minorities or those expressing unpopular views, or will sweep in reporting or commentary that is critical to public discourse. Apart from the difficulty of defining such a complex category, can an algorithm distinguish violent extremist content from commentary criticizing it? These concerns underscore why platform transparency is so important. A more robust accounting of YouTube’s practices would tell the public how frequently machine-flagged videos end up removed for each type of prohibited content. It would also disclose YouTube’s standards for defining categories like “violent extremist content.” Facebook has recently taken the step of disclosing the rules it applies in removing content, and YouTube should do the same.
YouTube’s transparency report raises other questions about the role of machine learning in content takedowns. In what circumstances do machines automatically remove content without any human review? Though the report emphasizes human review of flagged content, YouTube’s explainer video, “The Life of a Flag,” suggests otherwise:
We’ve developed powerful machine learning that detects content that may violate our policies and sends it for human review. In some cases, that same machine learning automatically takes an action, like removing spam videos.
Under what circumstances does YouTube’s machine-learning algorithm automatically remove videos flagged as potentially inappropriate? And how many videos have been removed without a human ever having reviewed them? We know that YouTube (via Google) partners with the Internet Watch Foundation, which identifies known child pornography images and gives them distinct “digital fingerprints,” or hashes. Social media companies then use the hashes to prevent the images from being posted. YouTube and others are adapting that approach to preempt the posting or sharing of violent extremist content. Setting aside the numerous questions about how content is deemed extremist and is selected for the hash-sharing effort, might YouTube be using other methods to automatically remove non-hashed content that has successfully been uploaded? The explainer video does not explain.
Lastly, the report does not grapple with a critical question underlying the platforms’ broader shift to machine learning. If machines are learning from human decisions, how are the companies ensuring that the machines do not reproduce, or even exacerbate, human biases? Whether in the context of predictive policing or the distribution of Medicaid benefits, we’ve consistently cautioned against relying too eagerly on machine learning, which may simply aggregate our biases and mechanize them. That risk seems particularly acute in the context of “violent extremism,” where human biases run deep. How is YouTube ensuring that its potent technology is not engaging in the same racial or religious profiling it may have learned from human reviewers?
WILL ARTIFICIAL INTELLIGENCE MAKE US LESS FREE?
There are no easy solutions. Companies like YouTube face government and public pressure to shut down content or be shut down themselves. Some companies are trying to develop nuanced ways to address the issue. Facebook, for instance, announced this week that it would implement an appeals process for removed content and released its internal guidelines for making content determinations. These are important changes, even if they don’t go far enough.
YouTube should clarify exactly how its takedown mechanisms work. Otherwise, we have no way to ensure the machines aren’t going too far.