Will ChatGPT Revolutionize Surveillance?
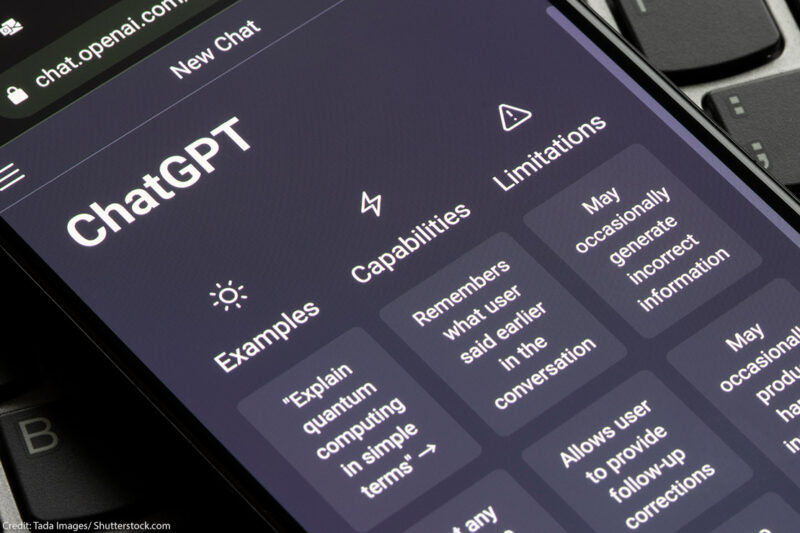
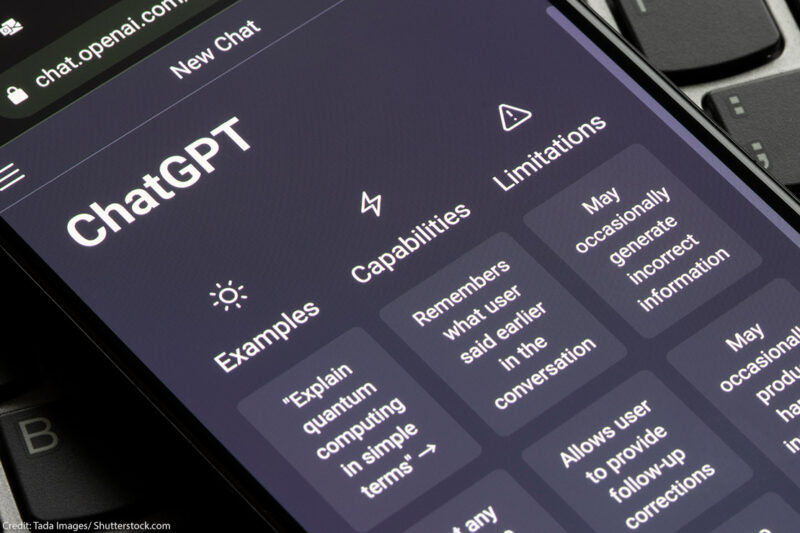
Everywhere you look lately, people are discussing the potential negative uses and consequences of the AI-driven chatbot ChatGPT. Many are concerned about the potential for ChatGPT and other “large language models” (LLMs) to spread a fog of disinformation throughout our discourse, and to absorb the racism and other biases that permeate our culture and reflect them back at us in authoritative-sounding ways that only serve to amplify them. There are privacy concerns around the data that these models ingest from the internet and from users, and even problems with the models “defaming” people.
But there’s another consequence of this technology that may prove to be very significant: its use as a tool for surveillance. If ChatGPT can “understand” complex questions and generate complex answers, it stands to reason that it may be able to understand much of what is said in a wiretap or other eavesdropped conversation, and flag particular conversations that are “suspicious” or otherwise of interest for humans to act upon. That, in turn, could lead to an enormous scaling up of the number of communications that are meaningfully monitored.
To get a feel for the possibilities here, I asked ChatGPT some questions.
A Rudimentary Test Run Talking to ChatGPT
To start off, I asked the model, “How suspicious is someone who says, ‘I really hate the president’?” ChatGPT answered, “It is not necessarily suspicious for someone to express dislike or hatred for a political figure, such as the president. People have varying opinions and beliefs about political leaders, and expressing them is a normal part of political discourse and free speech.”
So far, so good. “However,” it continued, “if the person’s statement is accompanied by specific and credible threats of harm or violence towards the president or others … then it may be cause for concern. In general, it’s important to consider the context and tone of the statement, as well as any accompanying behavior, before making a judgment about its level of suspicion or potential threat.”
Pretty good. Next, I gave ChatGPT a list of statements and told it to rate how suspicious each one was on a scale of 1-10. Though it again issued reasonable-sounding caveats, it dutifully complied with a table of results:
Even in this rudimentary little experiment we can see how a large language model (LLM) like ChatGPT can not only write, but can read and judge. The technology could be put to service as a lookout for statements that score highly by some measure — “suspiciousness” in my example, though one could attempt a variety of other monitoring projects, such as flagging “employees who are looking for a new job,” or “employees who have a positive attitude toward Edward Snowden.” (I ran a collection of published letters to the editor through ChatGPT, asking it to rate how positive each one was toward Snowden, and it was quite accurate.)
No Shortage of Potential Uses
There is a lot of demand for communications monitoring — by both government and the private sector, and covering not only private communications but public ones as well, such as social media posts.
In general, it is not constitutional for the government to monitor private communications without a warrant. Nor is it legal under our wiretapping laws for companies or individuals to do so. But there are plenty of exceptions. The National Security Agency collects communications en masse around the world, including, despite its putative foreign focus, vast amounts of internet traffic entering and exiting the United States including that of Americans. We believe this is unconstitutional, but our challenges have so far been dismissed on secrecy grounds. Companies also monitor private communications when carried out by their workers on work-owned devices. (Financial companies can be required to do so.) Prisons monitor inmates’ phone calls, and call centers record their customers (“This call may be monitored…”).
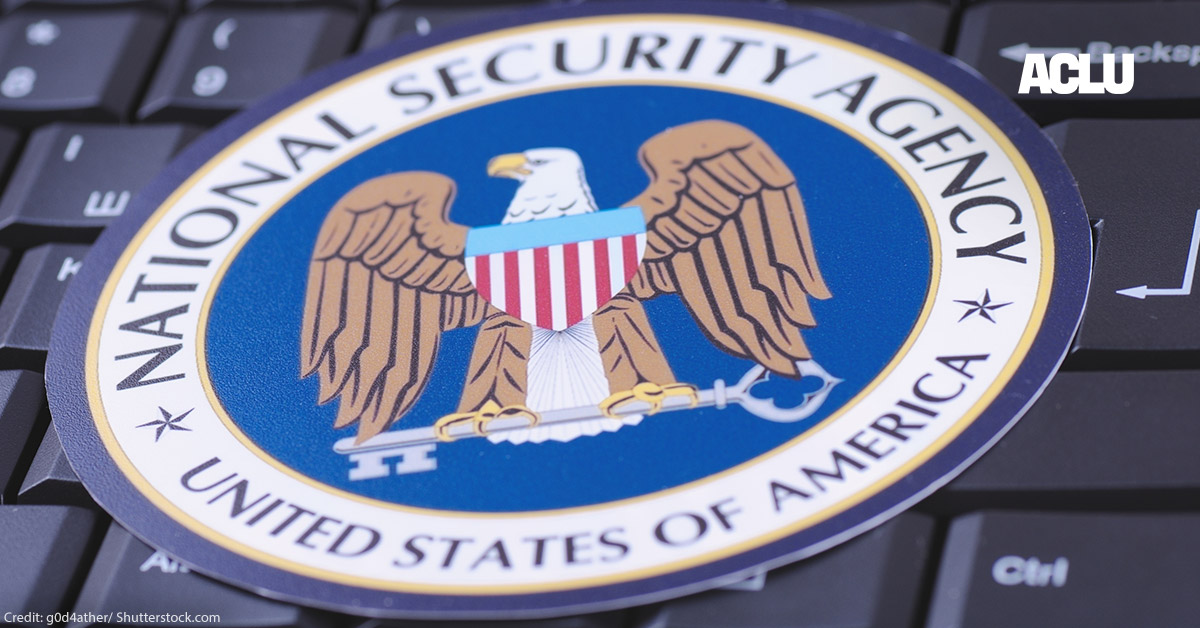
Five Things to Know About NSA Mass Surveillance and the Coming Fight in Congress
Congress must take this opportunity rein in the pervasive government surveillance enabled by Section 702.
Source: American Civil Liberties Union
When it comes to public communications, government agencies including the Department of Homeland Security and the FBI collect social media postings for wide-ranging purposes such as threat detection, the screening of travelers, and that perennial catch-all goal, “situational awareness.” Companies also sometimes search through their workers’ social media posts.
Currently, much of that monitoring is done through keyword searches, which flag the appearance of a particular word or words in a communications stream (aided in the case of oral conversations by rapidly improving speech-to-text engines). More sophisticated versions of keyword searches might look for the appearance of multiple words or their synonyms appearing near each other and try to use other surrounding words for context. Some corporate products for such monitoring claim to use “AI” (though that’s a typical marketing buzzword, and it’s often unclear what it means).
In any case, LLMs appear to have brought the potential for automated contextual understanding to a whole new level. We don’t know how sophisticated automated monitoring systems at the NSA have become, though in general, the private sector has often outpaced even the best-funded big government agencies when it comes to innovations like this. But even if the NSA already had some form of an LLM, this tool has now been brought into the open, and can clearly interpret language in far more sophisticated ways than previously possible for everybody else.
Accuracy and Unfairness Remain Core Concerns
The amazing performance of LLMs does not mean they will be accurate. My little experiment above shows that LLMs are likely to interpret statements that have perfectly innocent meanings — that refer to fiction or reflect sarcasm, hyperbole, or metaphor — as highly suspicious. More extensive experiments would have to be done to test the ability of an LLM to judge the suspiciousness of longer statements, but at the end of the day, these systems still work by stringing words together in patterns that reflect the oceans of data fed to them; what they lack is a mental model of the world, with all its complexities and nuance, which is necessary to properly interpret complex texts. They are likely to make big errors.
Some may argue that if LLMs are more sophisticated than something like a keyword scanner, that they will do less harm as eavesdroppers because of their greater ability to take account of context, which will make them better able to flag only conversations that are, in fact, truly suspicious.
But it’s not entirely clear whether more or fewer innocent people would be flagged as an AI eavesdropper gets smarter. It’s true that by recognizing context, LLMs may skip over many uses of keywords that would be reflexively flagged by even the most sophisticated keyword scanner. At the same time, however, they may also flag mundane words, such as “fertilizer” and “truck,” that might be ignored by a keyword scanner, but which in combination would be flagged because of LLMs’ greater sensitivity to context, such as a recognition that fertilizer can be used to make truck bombs, and a received belief that people with radical views are more likely to build such bombs.
In short, an LLM may make more sophisticated mistakes, but it may make just as many. And the very sophistication of the model’s judgments may lead human reviewers to take an AI warning much more seriously, perhaps subjecting the speaker to investigation and privacy invasions. The racism that the models absorb from the larger culture could also have very real-world consequences. Then there’s ChatGPT’s propensity for making stuff up; it’s unclear how that might play in.
But however effective or problematic LLMs prove to be as eavesdroppers’ aides — which is likely to vary by context — what we can be sure of is that all kinds of institutions are going to be trying it out.
A Question of Scale
Despite the unreliability of ChatGPT and its ilk, humans are also plenty capable of being erratic, ignorant of context, and generally stupid. The last statement in the above table was a 2012 tweet from a 26-year-old British man, Leigh Van Bryan, who was excited about his trip to Los Angeles with a friend. Upon arrival in Los Angeles the two were detained by Homeland Security, held in jail for 12 hours, and blocked from entering the United States despite their attempts to explain that “destroy” was British slang for “party in.” Van Bryan had also exuberantly tweeted that he was going to be “diggin’ Marilyn Monroe up” on Hollywood Boulevard (though she is not buried there), a reference to a line from the TV show “Family Guy.” Literal-minded federal agents searched the pair’s suitcases looking for shovels.
Regardless of relative intelligence levels, the biggest harm that might come from the use of LLMs in surveillance may simply be an expansion in the amount of surveillance that they bring about. Whether by humans or computers, attempts to interpret and search masses of communications are inevitably erratic and overbroad — we have already seen this in corporate social media content-regulation efforts. But if a lot more communications are being meaningfully monitored because humans perceive LLMs as better at it, many more people will be flagged and potentially hurt.
Hiring humans to review communications is expensive, and they’re distractible and easily bored, especially when required to pore over large amounts of ordinary activity looking for very rare events. If only as a matter of economics, AI agents would be able to ingest, scrutinize, and judge far more social media postings, emails, and audio transcripts than humans can do. Not only will that likely result in a higher volume of the kinds of monitoring that are already happening, but it will likely encourage an expansion in the parties that are doing it, and the purposes for which they do it. A company that has never considered monitoring its employees’ internet postings may decide to do so, for example, if it’s cheap and easy, and it doesn’t seem to generate too many false alarms. Or it might move from searching for signs of reputational damage to intelligence on which employees are thinking of leaving or are not dedicated to the company. Because why not? It’s all so easy to do. Any institution that thinks it can increase its power and control by using LLMs for surveillance, will likely do so.
No matter how smart LLMs may become, if they result in an expansion of surveillance — for purposes both serious and trivial — they will engage in far more misunderstandings and false alarms. And that, in turn, would create chilling effects that affect everyone. As stories of various institutions’ “successes” in flagging suspicious communications emerge — not to mention their mistakes — we would all begin to feel the growing presence of machines listening in. And, in certain contexts, begin to subtly or not-so-subtly censor ourselves lest we cause one of those AI minders to flag us. In this, LLMs may have the same effect with regard to communications that video analytics may have when it comes to video cameras.
We need to recognize that large-scale machine surveillance is likely coming our way, and whether the machines perform well or badly, better privacy laws will be vital to prevent powerful institutions from leveraging technology like LLMs to gain even more power over ordinary people, and to protect the values of privacy and free expression that we have always cherished.